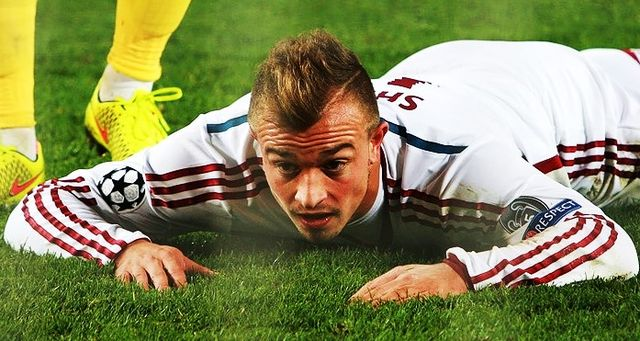
Image by Дмитрий Голубович. Source: Wikimedia commons
One of the interesting (but usually difficult) tasks regarding analysis of football matches by pundits and football lovers has to do with predicting the winner of a football match. This is because several factors come into play to determine the winner of a football match, be it deterministic factors such as the strength of teams or sometimes stochastic factors such as one-off events or an error in a football match. In this blog post, we seek to ascertain the strength of teams in the various football leagues across the world and provide a ranking according to their strength. Continue reading to get more insights.
What is the Problem?
Obviously, two teams in different leagues are not of equal strength. Can we compare the strength of Newcastle United (English Premiership) against VfL Bochum (Just promoted to Bundesliga 1)? Since these teams play in different leagues which may not be of equal strengths,
answering the above question is somehow complicated. There is some non-linearities or asymmetries in the strength of the leagues in which they operate; hence this complicates a direct like-for-like comparison between the two teams. As a first step, one could attempt to answer the above question by making some kind of educated guess. However, this becomes complex when the teams in question operate in different leagues at different divisions and may not hav
met in a competition in recent years or even not played opponents of comparable strengths.
The knowledge of the strengths of teams is significant for three main reasons: Firstly, it is quite difficult to analyse the game between opponents who hardly meet in a competition. It is also likely that such teams have not played similar opponents in a long time. So think about cup competitions such as the DFB-Pokal match between SV Wehen Wiesbaden (Bundesliga 3) versus Borussia Dortmund (Bundesliga 1) to be held in 2021/2022 season. The current strength of both teams if known would aid in the analysis of this particular match since it gives the basis for a direct comparison. Football pundits, analysts and football lovers can therefore tell which teams are the favourites and the underdogs in such football competitions. Secondly, consider a player transfer between different lower-tier teams in different countries. For instance player transfer from SV Wehen Wiesbaden (Bundesliga 3) to Swansea City (EFL Championship). How would a player involved in such a transfer fancy his performance in his new team?
Several factors come into play to determine the winner of a football match, be it deterministic factors such as the strength of teams or sometimes stochastic factors such as one-off events or an error in a football match.
A direct team ranking could provide a guide to the player regarding managing his performance expectations in his new team. Thirdly, the performance of teams over different periods in competitions is not static. That is to say that the performance of teams varies from season to season and from competition to competition. Getting some kind of index to measure and provide an updated strength of teams will provide sports stakeholders with the necessary tools to be able to make their day to day analysis and decisions. The above discussions highlight the significance of measuring the strength of teams, especially in different leagues or competition. In the subsequent sections, we will discuss a scientific approach to deal with the above question.
Our Approach to the Problem
The task is to measure the strength of teams across different competitions by way of ranking the teams in terms of their strength. Regarding the available datasets, Scoutpanel provides a rich source of data comprising about 600,000 matches played by about 13,000 teams all across the world in 2000 different competitions. Regarding the methodology, we apply the Glicko.2 rating algorithm, an improved variant of the Glicko rating system which is widely used for sports ratings. The variables used for the computation include teams initial team rating, a rating deviation that represents the standard deviation of the ratings and rating volatility which measures the degree of expected fluctuations in a teams rating. The initial ratings follows from the idea of the ”Elo” and ”Glicko” ratings where all teams are assigned an equal initial ratings of 1,500 points before the competition. The initial rating is discounted by a global league strength index for each league (1).
This ensures that the initial ratings of teams correspond to the strength of their respective leagues. Teams improve their ratings when they win against a much stronger opponent as compared to weaker opponent. Stronger opponents in the sense of other opponents with a high rating score than themselves and weaker opponents are those with a lower rating score. A team with high fluctuations in performance levels is assigned a high volatility index whereas a team with a consistent performance level earns a low volatility measure. In summary, the initial team rantings are used together with rating deviations and volatility index to compute updated ratings after match outcomes are recorded at the end of the period (football season). The updated rating deviations and volatilities indexes are also updated accordingly which serve as pre-information for the subsequent rating periods.
The highly ranked teams in descending order are FC Bayern Munich, Manchester City, Juventus, Inter Milan andFC Barcelona. Real Madrid, Liverpool and PSG
What now?
Let’s see how our team ranking results look like after applying the above-discussed methodology. Note that we focus on the first 20 ranked teams in the world. Unsurprisingly, these are all European teams as can be seen below. The highly ranked teams in descending order are FC Bayern Munich, Manchester City, Juventus, Inter Milan and FC Barcelona. Real Madrid, Liverpool and PSG follow in the list (Table 1). Is this result intuitive? Well, we observe that these teams have dominated their respective leagues and have been consistent in the champions league for the past seasons. Hence it is not surprising to see them dominate the ranking list. In addition to the rating index, we also generate a measure of the volatility of the ratings which depicts the consistency in the performance of the teams. Among the top 5 teams, it can be noticed that Bayern Munich has the most consistent performance. As a next step, we ascertain how our results compare with ratings by other football associations. UEFA provides a ranking of European clubs that is comparable to our results.
The UEFA team rankings are based on results obtained for all teams participating in European competitions. Hence teams which do not participate in UEFA competitions are not ranked. Figure 1 depicts the top 20 ranked teams by UEFA. Bayern Munich occupies the top spot followed by Real Madrid, Manchester City, FC Barcelona and Juventus in that order. Atletico Madrid, Man Utd, PSG, Liverpool and Arsenal complete the top 10 list.
[rev_slider alias=”glickouefa”][/rev_slider]
Comparing our rankings to that of UEFA, our result is more comprehensive because it also include ranking for all European teams which have not participated in UEFA competitions. Secondly, Arsenal, Manchester Utd and Atletico Madrid do not make our top 10 list. They are replaced by Inter Milan which won their domestic league competitions for the 2020/2021 season, and FC Porto and Atalanta which had a fine form in the Champions League. It can be concluded from our results that 7 out of the top 10 teams are similarly represented in the UEFA ranking list. Hence our results is comparable to the UEFA results. Additionally, our comprehensive result makes it possible to generate the ranking of all football teams on a country by country basis.
So how is the results from this paper useful to sports stakeholders? Knowing the strength of teams in terms of an index or ratings could serve as an input for making prediction about the winner of a match. For instance one could use rating information as an input for building further predictive model regarding the winner of a single match or competition. Secondly with this kind of information, it would be easier to compare the strength of teams across different leagues. Besides it gives some first hand information with respect to the strength of leagues in which these teams operate. Thirdly, knowing the strength of teams could form the basis for a further investigation regarding inequalities across the various leagues. So for instance one could look further to compare if the strength of teams are fairly or unequally distributed in each respective league. In order words, we could ascertain the competitiveness of leagues in which these teams operate once we have information about the strength of teams.